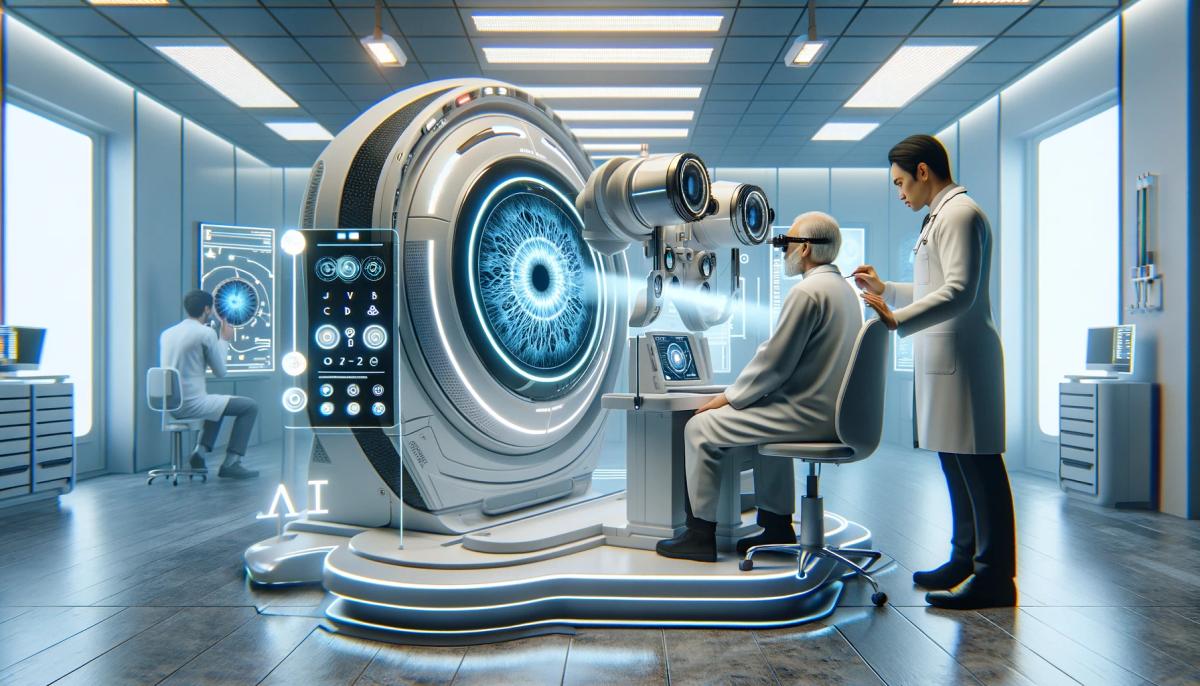
AI Ophthalmology Innovations - A New Era in Eye Care.
Key Highlights Artificial Intelligence and deep learning have revolutionized the field of ophthalmology by improving disease detection and diagnosis. ...
AI technology can assist in diagnosing diabetic retinopathy by analyzing retinal images for signs of the disease, such as microaneurysms or hemorrhages, with high accuracy. This helps in early detection and prompt treatment, potentially preventing vision loss in diabetic patients, and avoiding a global health crisis.
Diabetic retinopathy (DR) is a serious complication of diabetes that can lead to vision loss and blindness if left untreated. Early detection and treatment are crucial in preventing disease progression and preserving vision. However, there are significant challenges in screening and diagnosing DR, especially in underserved populations and remote areas where eye care is lacking due to access and education.
According to current guidelines by the American Academy of Ophthalmology, yearly screenings for DR are recommended for patients with diabetes to detect referable cases. These screenings include a vision and retinal examination, with DR severity graded according to a scale.
To aid in detecting and diagnosing DR, researchers have turned to technology, specifically Google Scholar, to access and analyze a wide range of studies and articles related to the topic. This tool has proven to be a valuable resource in advancing our understanding and management of this disease, particularly in underserved populations and those with limited access to eye care due to their diabetes mellitus diagnosis.
The above image shows a DR4 level fundus image, or a vision threatning DR.
The advent of Artificial Intelligence has revolutionized the field of DR detection. AI systems can accurately analyze and interpret retinal images, providing an efficient and reliable screening method for diabetic retinopathy. These Artificial Intelligence systems can detect early signs of the disease, enabling timely intervention and treatment for patients by assisting clinicians in identifying other diseases.
Integrating Diabetic Retinopathy AI detection marks a significant advancement in healthcare. By harnessing the power of AI technology, clinicians can now access efficient and accurate analysis of retinal images, enabling early detection of DR with Artificial Intelligence and subsequent intervention.
Through AI systems, the screening process for DR is streamlined, ensuring that patients receive timely treatment to prevent vision loss and blindness. This innovative approach enhances the quality of care provided and addresses the challenges faced in underserved communities where access to traditional eye care may be limited.
The following image shows one early detection of Diabetic Retinopathy with the help of Artelus' deep learning model. Early stages of DR (DR1) is represented by microaneurysms, which are very hard to detect as their sizes are extremely small. This stage of DR can be cured simply with diet control. But if ignored, it will only worsen and migrate to later stages of DR.
This technology has the potential to greatly benefit countries with a shortage of eye specialists, such as India and Thailand, by expanding high-quality DR screening programs. Additionally, this research has been published in JAMA, a leading medical journal with a high DOI (digital object identifier), providing further credibility for the effectiveness of diabetic retinopathy AI.
In this blog, we will explore how Artificial Intelligence is transforming the detection and management of DR. We will discuss the disease's global impact on vision health, the signs and symptoms to watch out for, and the role of AI in early detection. We will also delve into the advancements in AI technology and its impact on healthcare providers and patients. Lastly, we will explore AI's challenges and future directions in DR detection, real-world applications, and success stories in a diverse cohort of patients.
DR is a complication of diabetes that affects the blood vessels in the retina, the light-sensitive tissue at the back of the eye. High blood sugar levels can damage the blood vessels, leading to leakage and swelling. Over time, this can cause vision loss and, in severe cases, blindness.
There are different stages of DR, ranging from mild nonproliferative DR (NPDR) to severe proliferative DR (PDR). NPDR is characterized by microaneurysms and small areas of swelling in the blood vessels. PDR is characterized by the growth of abnormal blood vessels on the retina's surface, which can cause scarring and retinal detachment.
DRy often develops without any noticeable symptoms in the early stages. As the condition progresses, symptoms may include blurred vision, floaters, and difficulty seeing at night. Regular eye screenings are essential for early detection and timely treatment of DR.
DR is a common eye problem that greatly affects global vision health. It impacts many people and must be detected early for proper care. The International Diabetes Federation reports that 34 million individuals worldwide have DR. If not screened and treated promptly, it can lead to vision loss, including blindness, making it crucial to address the increasing number of people affected by this condition.
With more diabetes cases, DR increases too. AI tools help detect this eye problem early. They aid in finding the issue sooner and improving patient care by enabling quick action. AI boosts diagnostic abilities in healthcare, enhancing care for those at risk of this condition.
AI has revolutionized the healthcare field, particularly in the early detection of DR through the analysis of fundus images. By utilizing sophisticated algorithms and deep learning techniques, AI systems can efficiently identify abnormalities in the retina that may indicate DR. This technology enables rapid and precise analysis of vast quantities of images, equipping healthcare professionals with crucial information for timely interventions. However, it is important to note that the accuracy and effectiveness of these AI systems can be affected by insufficient-quality images, highlighting the need for continuous advancements in image quality algorithms.
Integrating AI into diabetic eye screening has exhibited significant promise in enhancing the accuracy and efficiency of disease detection. These systems aid healthcare providers in pinpointing patients who necessitate further evaluation and treatment, ultimately leading to improved outcomes for individuals with diabetes.
Diabetic Retinopathy AI streamlines the screening process and ensures patients receive timely care, potentially preventing DR-related complications.
Traditional screening methods for DR typically require ophthalmologists or optometrists to examine fundus images manually. However, with artificial intelligence (AI) advancements, diabetic eye screening has transformed thanks to automated detection processes.
AI-based systems can now analyze fundus images for indicators of DR, lessening the need for extensive manual review. These cutting-edge AI solutions have been integrated into medical devices utilized in primary care clinics and various healthcare settings.
Diabetic retinopathy AI screening has brought about notable enhancements in accuracy and efficiency. This progress has paved the way for early detection and timely intervention, ultimately improving patient outcomes and quality of care. By leveraging AI innovations, healthcare professionals can streamline the screening process, leading to more effective management of DR and its associated complications.
Artelus has made a significant impact in diabetic retinopathy AI screening, having screened an impressive 203,257 patients and identifying 48,000 cases of DR-positive instances. Founded and spearheaded by Mr. Pradeep Walia, their cutting-edge deep-learning models have played a pivotal role in the early detection of DR. It showcases their commitment to advancing healthcare technology. Artelus' results in AI advancements for Diabetic Retinopathy Screening earned them a spot in the prestigious Forbes magazine.
By leveraging Artificial Intelligence systems that analyze fundus images for DR indicators, Artelus has streamlined the screening process, leading to more efficient and accurate diagnoses. These deep learning algorithms have demonstrated exceptional accuracy in detecting DR, paving the way for timely interventions and treatment plans that can potentially save patients' vision.
Moreover, Artelus has successfully developed a method to compute the Cup-to-Disc ratio from a single fundus image, a crucial metric in assessing the risk of glaucoma. This innovative approach highlights Artelus's versatility and expertise in utilizing AI technologies for various eye diseases beyond just diabetic retinopathy. Artelus uses state of the art Attention based U-Net models for the Optic Disc and Cup segmentation and their proprietary algorithm thereafter to compute the Cup to Disc Ratio (CDR). If the CDR is greater than 0.5, then it is a primary Glaucoma suspect.
The following image shows that the model has predicted a CDR of 0.54.
DRISTi, which stands for Diabetic Retinopathy Intelligent Screening Technology Integrated, is an advanced medical technology designed to enhance the screening process for Diabetic Retinopathy (DR) among diabetic patients. This technology leverages the power of Artificial Intelligence (AI) to analyze retinal images with high precision and speed.
DRISTi's primary benefit is its capability to detect not only Diabetic Retinopathy but also 12 other retinal abnormalities such as AMD, Glaucoma, and more (as shown in the below report). Dristi also comes with an Explainable AI, that precisely highlights the regions of interest, and accelerates the whole diagnostic process.
Here's a detailed explanation of how DRISTi works:
DRISTi is built upon Artelus core Diabetic Retinopathy AI, a deep learning development platform specializing in creating complex and high-performance models for image analysis. This platform supports model deployment on both cloud and edge devices and includes tools to reduce data bias, thereby enhancing the generalization performance of the models.
The core model behind Dristi is a self-attention-based hybrid EfficientNet / Transformer / ConvNext architecture with multihead self-attention. However, unlike conventional implementations, our approach involves significant customizations to adapt the model for specific medical imaging tasks, including detecting eye pathologies such as diabetic retinopathy, macular edema, and glaucoma.
This ensemble model choice is motivated by its excellent accuracy and computational efficiency balance, which are crucial for processing high-resolution fundus images. The model's depth and width are scalable, which allows us to finely tune the network's capacity based on the complexity of our task and the computational resources available.
We integrate a custom multihead-attention mechanism to enhance the model's focus on relevant features within the fundus images. This module is designed to weigh regions of the image differently, allowing the model to pay more attention to areas that are more likely to contain pathological features.
This attention map processes features extracted by the base model, enhancing or dampening feature activations based on their relevance to the diagnostic task.
The model features multiple heads, each responsible for a different diagnostic output:
Each head is connected to the global average pooling layer of the base network, ensuring that spatial information is efficiently summarized before a prediction is made.
The input image is first processed by the base model and the attention module during the forward pass. The resulting feature maps are then pooled and passed through each classification head.
The outputs from each head are designed to handle different aspects of the disease detection task, from identifying specific features to assessing disease presence and severity. Loss functions are individually tailored for each output, combining categorical cross-entropy and mean squared error as appropriate, reflecting the distinct nature of each diagnostic category.
The described model architecture represents a sophisticated medical image analysis approach specifically tailored for detecting and classifying diabetic retinopathy and related eye diseases. We can significantly enhance the model's performance and utility in clinical settings by leveraging advanced neural network architectures like EfficientNetV2 or Vision Transformers and integrating custom modifications such as attention mechanisms and multiple specialized output heads. This approach improves diagnostic accuracy and aids in the early detection and treatment planning of diabetic retinopathy, ultimately contributing to better patient outcomes and integration with electronic health/medical records (EHR/EMR) systems.
First, let's look at a set of Real Fundus Images.
Now, let's look at a set of fake (synthetically generated) fundus images.
Magical! Aren't they?
Can you spot the difference?
Diabetic Retinopathy (DR), a major cause of preventable blindness globally, is often undiagnosed in its initial stage, known as DR1, due to the asymptomatic nature of microaneurysms. The scarcity of high-quality fundus images at this stage leads to insufficient training data, creating a cycle that hampers the development of effective AI detection models. This study presents a novel approach using StyleGAN3, an advanced Generative Adversarial Network, to generate synthetic DR1 images that could significantly enhance AI model training by providing a richer, more diverse dataset.
Our dataset comprised 2,602 high-quality DR1 images from various sources, including the Messidor Dataset, Kaggle's Diabetic Retinopathy Detection Dataset, and proprietary data collected using the Crystalvue NFC-600 Fundus Camera. The images were rigorously annotated, verified, and preprocessed to ensure consistency and quality, which are essential for effective model training.
StyleGAN3, developed by NVIDIA, represents a significant evolution in the GAN architecture, designed to produce high-fidelity images with minimized artifacts. Key to our approach is StyleGAN3's alias-free design and translation equivariance, which ensure that synthetic images retain critical diagnostic details such as the shape and texture of microaneurysms. Our implementation customized StyleGAN3's architecture to optimize it for the specific textural and geometric fidelity required for DR1 images.
The training protocol was meticulously designed to balance the optimization and performance of the generated images. We quantitatively assessed the model's output using advanced metrics such as Fréchet Inception Distance (FID) and Kernel Inception Distance (KID), along with rotation and translation equivariance tests. A Human Turing Test involving trained ophthalmologists was also conducted to evaluate the synthetic images' realism qualitatively.
The following plot shows the different model metrics as well as spectral analysis.
Our model achieved a final FID score of 17.29, indicating a high visual similarity to real DR1 images. The Human Turing Test results supported this, with a statistically significant ability of ophthalmologists to differentiate between real and synthetic images, underscoring the quality of the generated images. The KID score further confirmed the statistical similarity of the distributions of real and synthetic images.
The application of StyleGAN3 in generating DR1 images represents a significant advancement in diabetic retinopathy detection. The high fidelity of the synthetic images suggests that they can reliably supplement real images in training datasets, potentially transforming the landscape of diabetic retinopathy screening and prevention.
Spectral fidelity was assessed using Fast Fourier Transform (FFT) techniques, revealing that the synthetic images closely mimic the real images in the central frequency domain. However, some discrepancies were noted at the boundaries. These findings are crucial for confirming the clinical utility of synthetic images.
Bootstrap resampling methods were applied to the latter training stages to estimate the robustness of the FID scores, which consistently demonstrated the model's superior performance compared to traditional training datasets.
This research demonstrates the potential of using StyleGAN3 to create synthetic medical images that can significantly enhance the training datasets for AI models in ophthalmology. The ability to generate such high-quality synthetic images could revolutionize the early detection of DR by providing abundant, diverse, and accurate training data.
Looking ahead, we aim to expand this approach to other stages of DR and other ophthalmic diseases, potentially transforming the landscape of medical imaging and diagnosis. Integrating these synthetic images into routine clinical practice could lead to earlier and more accurate diagnoses, better patient outcomes, and reduced preventable blindness. Additionally, digital diagnostics, such as the IDx-DR program, have shown promising results in statistically validating diabetic retinopathy detection. This advancement in AI technology has the potential to greatly improve the future of detection and treatment for various medical conditions.
Using StyleGAN3 to generate synthetic DR1 images represents a promising advancement in AI in medical imaging. By augmenting the available training data, this method offers a viable solution to the data scarcity problem in early diabetic retinopathy detection, setting a new standard for developing diagnostic AI models.
Introducing high-quality synthetic images could revolutionize the training of Artificial Intelligence systems in ophthalmology, influencing future research directions and clinical practices. This approach promises to enhance early detection capabilities, potentially preventing blindness due to diabetic retinopathy.
This study demonstrated StyleGAN3's potential to create synthetic DR1 fundus images that can significantly enhance the training of Artificial Intelligence diagnostic models. By augmenting our dataset with synthetic images, we can improve the accuracy and reliability of diabetic retinopathy detection, offering a promising solution to the challenge posed by the scarcity of early-stage clinical images.
Diabetic Retinopathy AI detection tools employ sophisticated algorithms and machine learning methods. They are trained on extensive sets of fundus images, which are detailed retina photos. By analyzing features and patterns in these images, the AI algorithms can identify indicators of diabetic retinopathy.
Machine learning enables Artificial Intelligence models to enhance their accuracy through data-driven learning. The technology driving these tools is continuously advancing, and research and development are ongoing to improve their effectiveness and functionalities.
Artificial Intelligence studies retinal pictures to spot issues like DR. It finds lesions, irregularities, and signs of the condition. Models learn from many images to recognize patterns indicating the disease.
The quality of images significantly influences the accuracy of Diabetic Retinopathy AI interpretation. High-quality retinal images are essential for obtaining reliable results from Artificial Intelligence algorithms. Artificial Intelligence-generated insights from retinal images can provide healthcare professionals with crucial information to aid in the early detection and treatment of diabetic retinopathy.
Machine learning has advanced in healthcare, especially in finding DR. It helps diagnose, treat, and improve results. By using algorithms, it finds patterns in data that people may miss. Deep learning improved AI models' accuracy and reliability. These changes are revolutionizing healthcare by detecting issues early and improving patient care.
Diabetic Retinopathy AI has a considerable impact on healthcare providers and patients. By automating the screening process and reducing the time and effort needed for manual examination of retinal images, AI systems can support clinic staff in primary care clinics. This, in turn, streamlines patient encounters and enables healthcare providers to concentrate on treatment and management.
Using AI-based detection tools enhances the efficiency of diabetic retinopathy screening in primary care settings, resulting in earlier detection and intervention. Patients gain from Diabetic Retinopathy AI detection through timely and precise diagnoses, fostering appropriate treatment and better outcomes.
Diabetic Retinopathy AI-powered screening enhances accessibility and efficiency compared to traditional methods. Conventional screening techniques typically necessitate specialized equipment and knowledge, posing barriers to access in disadvantaged and distant regions.
Integrating AI screening tools into current healthcare systems expands the reach of retinal screening to primary care centers and other medical facilities. AI simplifies the screening procedure, cutting down on the time and resources needed to examine retinal images manually.
Diabetic retinopathy imposes a substantial strain on healthcare systems worldwide, necessitating resources for screening, diagnosis, and treatment. The utilization of AI for diabetic retinopathy detection alleviates this strain through enhanced screening accuracy. AI technology can swiftly and precisely analyze vast quantities of retinal images. So, it decreases the time and labor needed for manual inspection.
AI-powered detection tools can optimize healthcare resources and enhance the cost-effectiveness of diabetic retinopathy screening initiatives. The impact of diabetic retinopathy AI is significant. It helps healthcare handle more cases, reducing the pressure on healthcare workers.
AI is widely used in detecting diabetic retinopathy, but it faces challenges and future directions. Data privacy and security are crucial for creating Diabetic Retinopathy AI detection tools. Enhancing AI models' interpretability and addressing bias and fairness issues are future directions for diabetic retinopathy detection. Research and cooperation among healthcare providers, AI developers, and regulators are necessary for AI's progress and safe use in this field.
The prospect of incorporating AI into everyday healthcare practices is promising for detecting diabetic retinopathy. The utilization of AI has the potential to revolutionize the process of diabetic eye screening, enhancing efficiency and accessibility for a broader demographic. The future involves incorporating AI into electronic health records, telemedicine platforms, and primary care facilities to improve screening processes and boost patient results. Healthcare professionals stand to gain from AI's capacity to swiftly and accurately analyze extensive data, offering valuable insights for diagnosis and treatment.
Artificial Learning Systems India (ARTELUS) has been a beacon of innovation in healthcare technology, particularly in artificial intelligence (AI). The company's journey is marked by commendable achievements and recognitions that underscore its commitment to revolutionizing healthcare through cutting-edge technology.
Founded with the vision to democratize healthcare by leveraging AI, ARTELUS has been at the forefront of developing solutions that aid in the early detection of diseases. The company's focus on creating non-invasive screening tools has the potential to save millions of lives by catching diseases early when they are most treatable.
The success of ARTELUS has not gone unnoticed in the media, with over 50 publications featuring the company's work, including Forbes, Economic Times, and many more. This extensive coverage reflects the company's impact on the healthcare industry and its growing reputation as an innovator.
You can see all media release of Artelus here.ARTELUS's journey, spearheaded by its founder, Mr. Pradeep Walia is a shining example of how technology can make significant strides in healthcare when applied thoughtfully and innovatively. The company's success stories are not just milestones but stepping stones towards a future where AI and machine learning play a crucial role in saving lives and improving the quality of healthcare for people around the world. With a strong foundation and a clear vision, ARTELUS continues to push the boundaries of what's possible in AI and healthcare.
If you are interested to know more, or get an online demo, you can book an online call with Artelus' team here.
Global case studies have illustrated the efficacy of Diabetic Retinopathy AI screening. These studies reveal that AI-powered screening can enhance the precision and effectiveness of detecting diabetic retinopathy in various populations. Implementing AI systems enhances screening accessibility and minimizes care disparities. AI in diabetic retinopathy screening aids in timely identification, prompt intervention, and improved patient visual outcomes.
Patient feedback offers valuable insights into how AI-based screening for diabetic retinopathy positively impacts individuals' lives. Many patients credit early detection through AI for saving their vision and maintaining their eyesight. Satisfaction with AI screening is high due to its convenience, accuracy, and speed.
The accessibility of diabetic retinopathy screening through AI has empowered patients to manage their eye health effectively. Overall, patient testimonials emphasize the benefits of AI in diabetic retinopathy detection, supporting its integration into standard healthcare procedures.
AI is revolutionizing diabetic retinopathy detection, enhancing patient outcomes globally. Through AI innovations like DRISTi and GANs, early detection is more efficient and accurate, positively impacting healthcare providers. Integrating AI technology ensures accessible, efficient retinal screening, reducing burdens on healthcare systems. Despite challenges, ongoing advancements promise a future where AI-driven diagnostics are routine, fostering collaborations for global health initiatives. Real-world success stories and patient testimonials highlight the life-changing benefits of AI in diabetic retinopathy screening, paving the way for a brighter, tech-integrated healthcare future.
AI technology can assist in diagnosing diabetic retinopathy by analyzing retinal images for signs of the disease, such as microaneurysms or hemorrhages, with high accuracy. This helps in early detection and prompt treatment, potentially preventing vision loss in diabetic patients.
Key Highlights Artificial Intelligence and deep learning have revolutionized the field of ophthalmology by improving disease detection and diagnosis. ...
IntroductionArtificial Intelligence (AI) in Healthcare has already been used in radiology and drug discovery. However, many other healthcare areas cou...
Key Highlights AI is revolutionizing the healthcare industry, with the AI healthcare market projected to be worth $187 billion by 2030. AI technologi...
# From Pandemic to Endemic - The new NormalFor a world grappling with the new coronavirus, it’s becoming increasingly clear that even when the pandem...
The foundational ethos of Artelus is encapsulated in its forward-thinking vision: Leveraging state-of-the-art Deep Learning architectures to democratize healthcare for the "Forgotten Billions". At Artelus.ai, we are dedicated to advancing eye care through innovative technology and comprehensive solutions, making high-quality diagnostics accessible to all. Join us in revolutionizing healthcare and improving the lives of millions around the world.