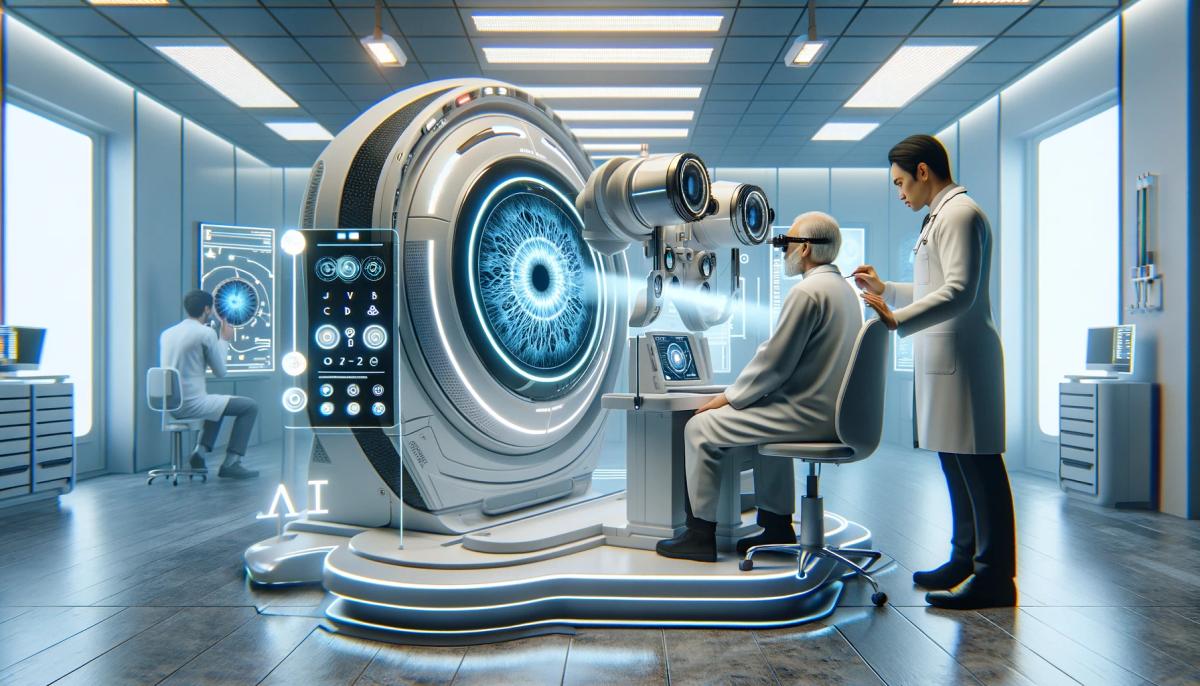
AI Ophthalmology Innovations - A New Era in Eye Care.
Key Highlights Artificial Intelligence and deep learning have revolutionized the field of ophthalmology by improving disease detection and diagnosis....
Artificial Intelligence (AI) in Healthcare has already been used in radiology and drug discovery. However, many other healthcare areas could benefit from AI applications. This article explores how AI in healthcare can improve it with examples.
Still, this approach is often limited by doctors' ability to interpret a patient's symptoms and possible outcomes. AI could, therefore, be useful in shrinking the time it takes for diagnoses using laboratory testing. This reduced time is precious when these tests may have life-saving implications for patients.
A practical application that could benefit patients would be oncology research, particularly gene sequencing technologies like high throughput parallel microarray analysis(HTA). The more accurate a test is at determining the outcome of an illness, the faster it is to make life-saving decisions. The wide range of applications for artificial intelligence makes it difficult to ensure ethical behavior. Given its ability to emulate and create simulations so realistic, decisions may be eliminated during tests on humans if widespread within the healthcare industry. So, we could replace top doctors with machine-based doctors even when human factors are operational.
However, this is only one of the many ethical dilemmas that will be faced in the future. Artificial intelligence development and its use in healthcare may bring more issues to light than solve. In the end, we are still a long way from having a fully functional artificial intelligence system to replace doctors, but it is an exciting time for those who study these new technologies.
In this article, we will discuss some recent advancements in artificial intelligence (AI) in healthcare and their implications.
Artificial Intelligence (AI) is a machine's ability to mimic human cognitive functions. Healthcare uses AI to improve medical care efficiency and improve health outcomes. Here are some examples of AI in healthcare:
Natural Language Processing (NLP) is a branch of ML that tries to understand what people are saying by understanding language. This field has been advancing at an accelerated pace, with more and more novel systems that can help better the world. For example, we have seen many achievements through natural-language processing technologies like predictive text input for mobile keyboards, etc. NLP and ML are helpful in healthcare because they can help people who need different types of care, from diagnoses to monitoring a patient's condition. In addition, natural-language-based systems can personalize health records without any human intervention.
Machine Learning is a branch of AI that provides computers with knowledge. Unlike human intelligence, which requires constant reinforcement and new information. These machines can filter through vast amounts of data, make predictions based on contexts, and understand their relationships. The contexts can be age, location, gender, or other variables describing an individual.
Deep learning has been in the media for a considerable amount of time. Still, it was only recently that its reach expanded beyond specific fields such as computer vision and natural language processing. When using AI for healthcare, deep learning is essential, making sense, as AI heavily relies on machine-learning algorithms. An algorithm built through deep learning can be concerned with more complex tasks.
Also, deep learning can detect patterns and relationships in reams upon reams of data, which is crucial for healthcare scenarios. Doctors analyze large amounts of information daily in healthcare while considering multiple variables each patient presents.
DNNs are artificial intelligence algorithms that can process a large amount of data. For example, they may become part of computer programs to learn how language works and use it in associative learning processes. This learning method is crucial for applying AI to healthcare since diseases vary drastically between patients. Hence, an ML algorithm must decide which treatments would work best based on each patient's characteristics such as age, gender, etc.
There are several deep-learning frameworks that the healthcare industry can use. Google Brain---a tool to help developers create intelligent systems---is an example, while Tensorflow is another one worth mentioning. Neural networks used in healthcare have also entered various industries, including healthcare sectors such as radiology, pathology, and pharmacology.
Reinforcement learning (RL) is another subfield of artificial intelligence. RL focuses on simulating an agent's behavior based on its experiences, with rewards attached to actions. For example, it teaches how a robot should move in response to what it sees or hears.
The mechanisms used here are similar to those suggested by theories like Goodhart's law and Generalized Trust Criteria (GTC). Embedded heavily in healthcare, reinforcement learning is used through various devices such as mobile phones to encourage healthy behavior.
A fuzzy logic system allows computer systems to take fuzzy inputs. These inputs can range from environmental conditions such as weather or health data to a set of rules, which could include human guidance. A fuzzy logic system produces an output that is more complex or less predictable than the one made by simple regulations alone. A deeper investigation into how algorithms learn through simulations on large datasets has helped achieve better results and provided insights into phenomena in the social and biological worlds.
Medical robots are used in healthcare to improve the treatment of diseases. These robots can perform precise movements, which allows them to move around the body straightforwardly. This way, they can treat the affected area without causing any damage to it. Medical robots are highly accurate and do not cause any pain or discomfort to the patient. Their main benefit is that they help diagnose and treat the disease without causing pain or discomfort.
Robotic process automation uses advanced robotics, computers, and software to automate tasks that humans previously carried out. It helps healthcare providers faster processing, more accurate results, and more secure environments. This technology can eliminate repetitive manual tasks, improve efficiency and enhance security within the healthcare industry.
Robotics in healthcare systems has increased with time due to the increased patient population worldwide, which needs urgent attention. It addresses orphan diseases that are often difficult for human physicians. One challenge is the increasing amount of clinical data to analyze and extract actionable insights. Healthcare organizations can deploy robotics into IT infrastructure to automate manual processes that took hours or days previously. It required several people in cognitively intensive labor, consuming many financial resources. Robotic systems directly contribute to lowering human productivity.
It achieves this ease by:
As a result, RPA technologies integrate with existing IT infrastructure without disrupting change or damaging computer systems.
Significant development has occurred in artificial intelligence research in the last decade. New systems aim to use AI for practical applications and help reduce human error related to clinical decision-making through automation.
Artificial Intelligence is a powerful engineering tool that completely streamlines workflows. It speeds up the process of diagnosing diseases requiring multiple steps or procedures by reducing errors caused by physician inconsistency between diagnosis, treatment, and performance. In this article, we discuss some major examples of AI in healthcare.
This is a major example of AI in healthcare. Cancer-related diagnoses demand more than the trained hands of experienced specialists. Predictions that come from AI systems lead to higher quality and better performance in cancer diagnosis, with little human intervention.
Researchers have already started developing patient record data into digital diagnostic tools. These tools leverage a computational platform called Deep Learning for Vision, introduced by NVIDIA, Inc. This technology seeks classification problems, incorporating new image recognition approaches. It solves this by augmenting them and thereby producing higher overall results.
Patient records can now be analyzed by artificial intelligence to implement new diagnoses. This results in alterations or operations that are not readily obvious to even the most experienced physicians. Advances in AI and observation have created a framework to determine all diagnosis possibilities before the operating room intervention. These frameworks drastically reduce the complications found within tumor sites when they become visible.
Artelus's DRISTi, a standout example of AI in healthcare, revolutionizes the detection of diabetic retinopathy by employing advanced AI algorithms. This innovative tool analyzes retinal images to identify early signs of diabetic retinopathy, a leading cause of blindness in diabetic patients. A company founded by Mr. Pradeep Walia, Artelus' DRISTi's strength lies in its precision and ability to assess the severity of retinal changes. It offers healthcare professionals a robust diagnostic tool that speeds up the evaluation process and enhances the accuracy of diagnoses.
DRISTi's AI-driven system simplifies the interaction between healthcare providers and patients by providing clear, actionable insights into the patient's retinal health. With its real-time data processing capabilities, DRISTi can adapt to new findings instantaneously, improving the diagnostic workflow and facilitating more effective treatment plans. This dynamic adjustment is crucial for tailoring patient-specific management strategies, ensuring that each patient receives the most appropriate intervention based on their unique condition.
Furthermore, DRISTi enhances patient engagement by allowing them to better understand their own health data. This feature empowers patients to actively participate in their healthcare journey, fostering better communication with their providers. By integrating patient feedback directly into the system, DRISTi continuously refines its diagnostic algorithms, thereby driving advancements in treating diabetic retinopathy.
The next example of AI used in Healthcare is earlier Cancer Detection. Some research labs are working to bring back more success to AI for Cancer Molecular Detection. They are studying cases and experiences of patients who are willing to get their tumors checked. They realized two possible classification systems in paraffin blocks by combining extraction with machine learning: Text Classifier and Computer Vision Histology Classification Systems. The former method helped one discern whether or not a particular area of a tumor was benign or malignant. At the same time, the latter classifies pieces of tissue into various disease types.
These features alone enabled the improvement of Cancer MDS using Deep Learning Algorithms to diagnose these cancers with such high accuracy and understanding, leading them towards furthering its applications for other glands within both medical and scientific fields. The only problem that remained was how it would inform both surgeons in preparing patients for surgery and how automated systems would primarily affect the lifespan of their platforms.
To help answer these questions, they began looking at other issues that could arise during this process. One such issue is false positives, False negatives, and unusual accuracy rates sometimes arise when using the Deep Learning Algorithm on digitized images. These results mainly occurred between those samples evaluated with Deep learning classification and those in paraffin blocks.
A Deep learning algorithm achieved this makeover. It was adapted further to cope with other diseases like sarcomas or muscle regeneration. This breakthrough increased their applications across biomedical and bioinformatics research fields.
The next example of AI in healthcare is diagnosing deadly blood diseases, and it is effective. Information technology has helped improve the diagnosis of blood diseases like leukemia, lymphoma, and sickle cell disease. Artificial intelligence has helped diagnose these deadly blood diseases by analyzing the patient's genetic information. In the article on a study done in 2021, artificial intelligence helps make vital decisions about patients with sickle cell disease. It improves the quality of life of people with these chronic diseases and their families and maximizes patient experience by providing more accurate and reliable information for them.
The research says that AI has successfully overcome several barriers to recognizing critical features of blood vessels, such as patterns such as vessel geometry, and branching. Another study declares that AI can handle investigations in projects related to acute leukemias, not only due to its potential for image-based diagnosis but also directly by providing vital information regarding oncogenic mutations.
AI is vital in modern radiology due to its true-to-life imaging capabilities. IBM has developed the AI platform that could help cancer radiologists by analyzing multidimensional images, which reenacts x-ray and CT scans of patients to improve upon radiological practice guidelines and increase accuracy. In addition, AI helps detect abnormality more accurately than human specialists can.
The AI software triggers when an X-ray film or CT scan compares two objects to each other, is scanned for abnormalities detected in various parts of it, and compares MRI images taken from past studies. Using such data can apply weighted robotic tools to help doctors make judgments and comparisons for the difference of patterns seen according to different radiographic studies. This is a crucial example of AI being used in healthcare.
Detecting problems like cancerous cells missed by human specialists, such as colonoscopies, possibly using 3D medical imaging. The machine learning algorithm elaborated on this technology is powered by a deep-learning approach. Deep Learning uses pattern recognition techniques from biology and recent advancements in the cognitive science field combined with other computer engineering disciplines. As a result, it can identify images of disease and intervention from many similar molecular structures in x-ray photography.
Artificial Intelligence (AI) is being used to develop new medicines. AI uses a computer to mimic the human brain. It is a way of using computers to make decisions. AI can help predict how a drug will work in humans and interact with other medications. AI can also help determine which patients respond best to a particular medicine. The AI can also predict side effects and monitor how the drug works in patients. Predicting what drugs will likely work best often requires a computer to "learn" by watching how patients react. These systems use millions of instances and carefully produced information from human experts (such as doctors, pharmacists, and nurses), laboratory results on drug interactions, and chemical responses in organisms (sea urchins). These predictions are verified using animals or humans for experiments before the suggested action gets implemented in practice.
The development of new medicines is often a lengthy and costly process. There are many different stages, each requiring careful management. AI in biopharmaceutical development is an interdisciplinary trend that facilitates the translation of scientific insights into the generation of new pharmaceuticals. More generally, AI-based methods help in the prediction using big data sets. The systems then integrate these into computer simulations. This is the next example of AI in healthcare.
The initial focus was cancer drug discovery, where computational methods were employed to assess efficacy rates without animal experiments. The first biological applications developed due to AI-enabled drug discovery include tests on early lead compounds against targets such as DNA and protein binding. In addition to other benefits in accelerating product development, implementing these methods can also reduce animal use due to the reduction in the number of animals used per target solution.
The next example of AI in healthcare is helping the medical field detect rare diseases. It helps medical professionals in finding rare diseases. AI has been able to detect a sporadic disease called Pelizaeus-Merzbacher disease. This disease is only present in Europe, and it has been detected in only two cases. More so, in the human medical resources of hospitals, AI can also detect rare diseases, as stated above. This detection is possible by looking at family health over generations. With this, they can find rare diseases by detecting linked gene mutations.
The pharmaceutical industry is developing new approaches to drug discovery, but many of these are highly experimental. Technology and data analytics are helping researchers overcome the challenges of biology and find new ways to discover novel medicines.
Artificial Intelligence (AI) is an essential aspect of digital drug discovery. AI can find new compounds that can cure or treat diseases. AI helps identify potential drug targets and can identify the best candidate compounds from a large set of compounds. Image Classification is used in drug discovery due to the enormous spectral differences caused by chemical constituents from certain drugs.
AI can automate this process, significantly reducing the sample analysis time and providing consistency with pre-existing methodologies for toxicological testing of new compounds. Additionally, digital robotic chemistry gained a higher accuracy at discovering novel antiaromatic than single workflows without them; there was also more certainty in the outcomes.
Clinical trials are research studies that test the safety and effectiveness of new medical treatments. There are many types of clinical trials, including phase I, II, and III clinical trials and randomized controlled trials (RCTs). These differ in the kind of research participants that take part in them. Phase I trials evaluate a drug's potential for treating cancer or other diseases. Phase II trials compare two drugs to determine which is more effective than the other.
Neural networks are the core of AI-based algorithms used in clinical trials. These algorithms help analyze a patient's medical history and predict the probability of disease. This helps to make an accurate decision about whether or not to treat a patient with a particular drug.
One of the main hurdles in drug development is selecting the right patient for a drug that millions of people will use. Deep Genomics uses cutting-edge machine learning algorithms to select patients most likely to respond to experimental treatments. The system analyzes and compares thousands of factors, including genetic data, physical and mental health conditions, lifestyle habits, etc. The algorithm then uses its findings to predict the likelihood of each patient's response.
AI is already improving healthcare by diagnosing medical conditions, providing on-demand health services, and making treatment decisions. However, as AI gets more advanced, it can begin to automate entire patient experiences. That could lead to a significant improvement in how people interact with the health system.
Patient flow optimization is one of the most promising areas of medical technology. It combines patient care, information technology, and artificial intelligence to improve the efficiency of clinical tasks. Patients' needs continuously change, and the demand for their services fluctuates throughout the day. Different areas of the country may have additional requirements. Like in real estate, there is a demand and supply indexes. Since various patients will use the system, they will likely have varying expectations regarding treatment times, the number of communications received, and the number of visits required.
AI practitioners can use patient flow optimization software to facilitate better efficiency while maximizing standardized outcomes across time. They can optimize this through sophisticated analytics on-demand visit calculations using all data, prioritizing workflow, and reducing doctor/patient interactions to improve overall patient outcomes.
AI is already creating revolutions in healthcare, and several companies are now providing it to patients. One of the most advanced AI-assisted surgical platforms in the world is Medrobotics. Medrobotics offers the world's first steerable, shapeable, endoluminal flexible robotic system to provide ScarfreeTM head, neck, and colorectal procedures. The Flex® Robotic System is intended for robot-assisted visualization and surgical site access in the throat, anus, rectum, and distal colon. It was developed based on algorithms designed explicitly for the unique anatomy of these areas, and it can also offer precision in complex surgical procedures like colorectomies.
The AI technology used by Medrobotics improves spinal cord injury surgeries with high accuracy and zero downtime, thereby providing a significant benefit over human surgeons during expensive surgeries that pose more risks. The operating table utilized by the robotic systems can even be adjusted according to different patients' needs. In addition, Medrobotics enables the visualization and control of medical robots in real time during surgery. Thus, it reduces interventional timesaving surgeries that are sometimes performed to more than 98%.
AI is playing a huge role in the healthcare industry, and it has many benefits. There are still challenges for the future of AI in healthcare, but the field is moving forward rapidly, and we will certainly see more innovation in this area soon. AI has many benefits for the healthcare industry. In this paper, I have discussed some of those benefits. One is to reduce human participation and work hours in procedures by 85%. A second one is to speed up treatments due to increased computing power during such procedures. Lastly, errors will be reduced thanks to the ability to pick up on patterns or patterns it sees over time.
The most popular AI platforms used in healthcare include IBM Watson, Google Cloud Healthcare API, Microsoft Azure Healthcare Bot, and NVIDIA Clara. These platforms leverage artificial intelligence to streamline healthcare processes, improve patient care, and enhance medical research.
Key Highlights Artificial Intelligence and deep learning have revolutionized the field of ophthalmology by improving disease detection and diagnosis....
AI technology assists in diagnosing diabetic retinopathy.AI technology can assist in diagnosing diabetic retinopathy by analyzing retinal images for ...
Key Highlights AI is revolutionizing the healthcare industry, with the AI healthcare market projected to be worth $187 billion by 2030. AI technolog...
# From Pandemic to Endemic - The new NormalFor a world grappling with the new coronavirus, it’s becoming increasingly clear that even when the pandem...
The foundational ethos of Artelus is encapsulated in its forward-thinking vision: Leveraging state-of-the-art Deep Learning architectures to democratize healthcare for the "Forgotten Billions". At Artelus.ai, we are dedicated to advancing eye care through innovative technology and comprehensive solutions, making high-quality diagnostics accessible to all. Join us in revolutionizing healthcare and improving the lives of millions around the world.